Book Downloads Hub Reads Ebooks Online eBook Librarys Digital Books Store Download Book Pdfs Bookworm Downloads Free Books Downloads Epub Book Collection Pdf Book Vault Read and Download Books Open Source Book Library Best Book Downloads Ursula K Le Guin Michael L Straley Colin Barrett Brigid Holder Maria Pretzler Carlos G Tutor Yelena Bailey Mark Urban
Do you want to contribute by writing guest posts on this blog?
Please contact us and send us a resume of previous articles that you have written.
Unlocking the Power of Hebbian Learning And Negative Feedback Networks: Advanced Information And

Are you ready to delve into the world of cutting-edge technologies and advanced machine learning algorithms? Brace yourself for an exciting journey as we explore the concepts of Hebbian Learning and Negative Feedback Networks.
The Fascinating World of Hebbian Learning
Hebbian Learning, named after the Canadian psychologist Donald Hebb, is a prominent theory in the field of neuroscience and artificial intelligence. It revolves around the idea that when two neurons repeatedly coactivate, the connection between them strengthens as a result. This synaptic plasticity is the foundation of learning and memory in the human brain.
In the context of artificial neural networks, Hebbian Learning provides a mechanism for the networks to learn and adapt based on input patterns. As the input data is presented to the network, the connections between the neurons are adjusted to optimize the overall performance and improve the ability to recognize patterns and make predictions.
5 out of 5
Language | : | English |
File size | : | 5786 KB |
Text-to-Speech | : | Enabled |
Print length | : | 401 pages |
Imagine a scenario where a convolutional neural network is trained to recognize different objects in images. Through Hebbian Learning, the network can reinforce the connections between neurons that fire in response to specific visual features, enhancing its capacity to accurately identify objects in future instances.
Unraveling Negative Feedback Networks
While Hebbian Learning strengthens connections, Negative Feedback Networks introduce a corrective mechanism that enhances the stability and efficiency of the learning process.
Negative feedback refers to the process of feeding the error or the difference between the desired output and the actual output back into the system to adjust its behavior. In the context of machine learning, negative feedback networks use this mechanism to refine the network's predictions and reduce the occurrence of errors.
By incorporating negative feedback, the network can continuously learn from its mistakes and improve its accuracy over time. In essence, it helps the network to fine-tune its parameters and weights, ultimately achieving better performance and higher reliability.
The Synergy of Hebbian Learning and Negative Feedback Networks
Now, let's explore the powerful synergy between Hebbian Learning and Negative Feedback Networks in the context of advanced information processing.
When combined, Hebbian Learning and Negative Feedback Networks create a dynamic learning system capable of adapting to complex and ever-changing environments. The Hebbian Learning component ensures that the network can identify and remember relevant patterns, while the Negative Feedback mechanism refines the network's predictions based on the feedback received.
This synergy is particularly relevant in applications such as speech recognition, natural language processing, autonomous vehicles, and robotics. For example, in autonomous driving systems, the network can continuously learn from its experience on the road, adjusting its behavior based on negative feedback from potential errors or hazardous situations.
Benefits and Advancements
The utilization of Hebbian Learning and Negative Feedback Networks brings several significant benefits and advancements to the field of machine learning and artificial intelligence.
- Increased adaptability: The network can quickly adapt to changes in the environment or input data, ensuring its performance remains optimal in dynamic scenarios.
- Enhanced accuracy: Through continuous learning and feedback, the network can improve its predictive capabilities, resulting in higher accuracy and reliability.
- Improved stability: Negative feedback helps in stabilizing the learning process, reducing the occurrence of errors and allowing for smoother and more consistent learning.
- Efficient resource utilization: The combination of Hebbian Learning and Negative Feedback Networks allows the network to optimize its resources and prioritize the most important patterns, leading to improved efficiency.
- Real-time adaptation: The adaptive nature of Hebbian Learning and Negative Feedback Networks makes them suitable for real-time applications where immediate adjustments are crucial.
Challenges and Future Potential
While Hebbian Learning and Negative Feedback Networks have shown great promise, there are still challenges that researchers and developers need to address.
One significant challenge is the interpretability of the learned representations. As these models become more complex and sophisticated, deciphering the exact decision-making process of the network becomes increasingly difficult. Efforts are being made to develop interpretability techniques to shed light on the inner workings of these advanced networks.
Looking ahead, the future potential of Hebbian Learning and Negative Feedback Networks is vast. As researchers continue to refine and optimize these algorithms, we can expect significant advancements in areas like personalized medicine, predictive analytics, and intelligent systems that better understand, adapt, and interact with humans.
Hebbian Learning and Negative Feedback Networks represent the cutting edge of machine learning and artificial intelligence. These advanced techniques offer exciting possibilities for various industries and applications, enabling systems to continuously learn, adapt, and improve in complex environments.
As our understanding of the human brain and information processing deepens, the incorporation of these advanced algorithms will undoubtedly contribute to the development of more intelligent and capable systems, revolutionizing the way we interact with technology.
So, fasten your seatbelt and get ready to witness the future unfold with Hebbian Learning and Negative Feedback Networks!
5 out of 5
Language | : | English |
File size | : | 5786 KB |
Text-to-Speech | : | Enabled |
Print length | : | 401 pages |
This book is the outcome of a decade’s research into a speci?c architecture and associated learning mechanism for an arti?cial neural network: the - chitecture involves negative feedback and the learning mechanism is simple Hebbian learning. The research began with my own thesis at the University of Strathclyde, Scotland, under Professor Douglas McGregor which culminated with me being awarded a PhD in 1995 [52], the title of which was “Negative Feedback as an Organising Principle for Arti?cial Neural Networks”. Naturally enough, having established this theme, when I began to sup- vise PhD students of my own, we continued to develop this concept and this book owes much to the research and theses of these students at the Applied Computational Intelligence Research Unit in the University of Paisley. Thus we discuss work from • Dr. Darryl Charles [24] in Chapter 5. • Dr. Stephen McGlinchey [127] in Chapter 7. • Dr. Donald MacDonald [121] in Chapters 6 and 8. • Dr. Emilio Corchado [29] in Chapter 8. We brie?y discuss one simulation from the thesis of Dr. Mark Girolami [58] in Chapter 6 but do not discuss any of the rest of his thesis since it has already appeared in book form [59]. We also must credit Cesar Garcia Osorio, a current PhD student, for the comparative study of the two Exploratory Projection Pursuit networks in Chapter 8. All of Chapters 3 to 8 deal with single stream arti?cial neural networks.
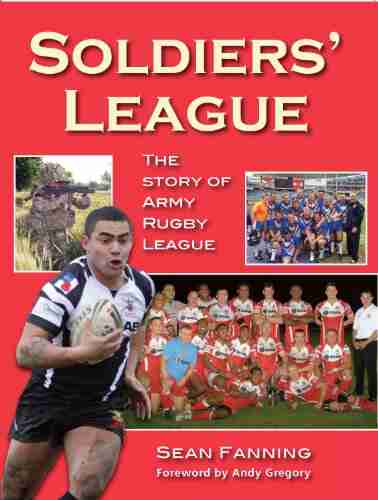

Soldiers League: The Story of Army Rugby League
The Origin and History The Soldiers...
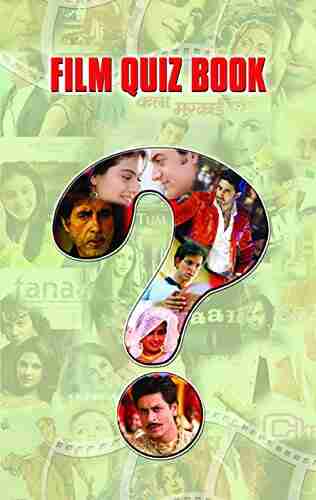

Film Quiz Francesco - Test Your Movie Knowledge!
Are you a true movie buff? Do you...
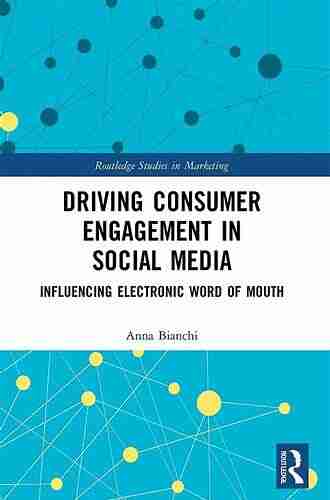

Driving Consumer Engagement In Social Media
: Social media has...
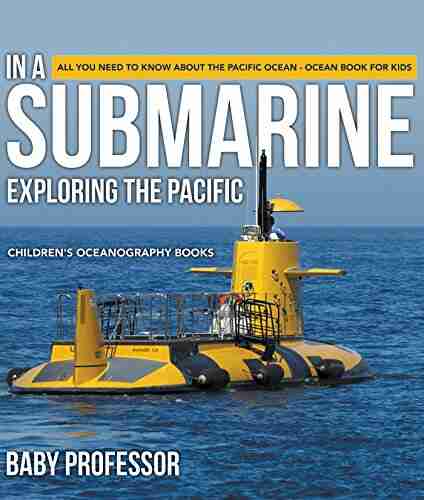

All You Need To Know About The Pacific Ocean Ocean For...
The Pacific Ocean is the largest ocean in...
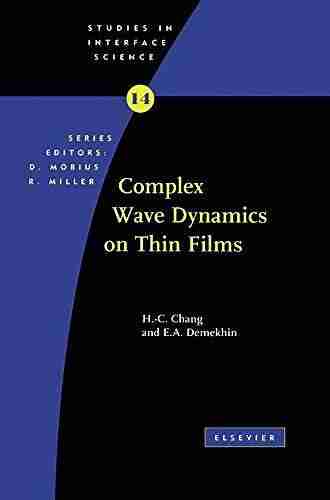

Unveiling the Intriguing World of Complex Wave Dynamics...
The study of complex wave...
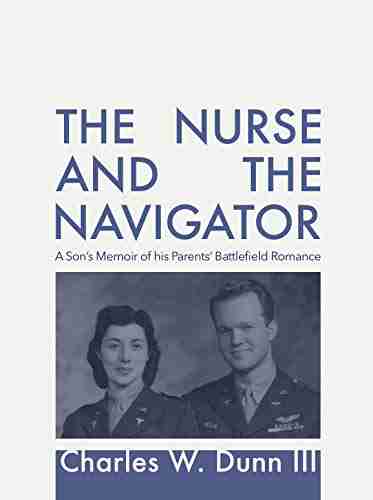

Unraveling the Mysterious Journey of "The Nurse And The...
Once upon a time, in a world of endless...
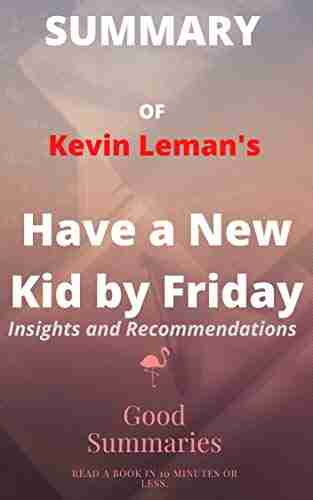

How To Change Your Child's Attitude and Behavior in Days
Parenting can be both challenging and...
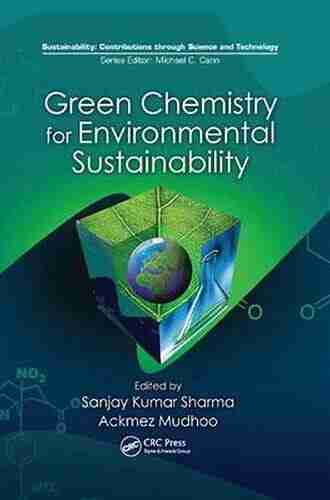

10 Groundbreaking Contributions Through Science And...
Science and technology have always...
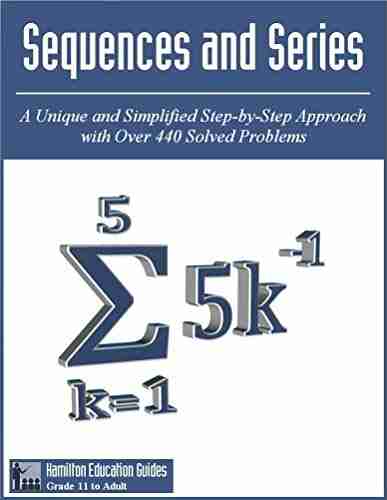

Unleashing the Power of Hamilton Education Guides Manual...
Are you struggling with understanding...
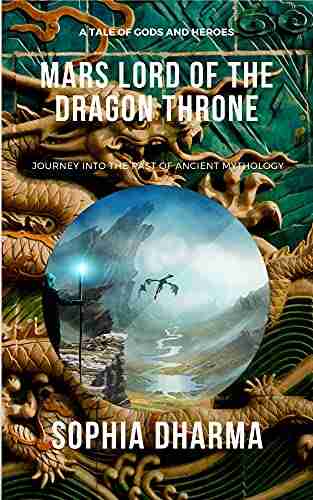

The Astonishing Tale of Mars: Lord of the Dragon Throne -...
There has always been a remarkable...
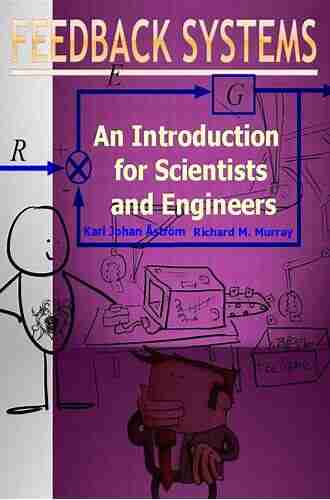

An Introduction For Scientists And Engineers Second...
Are you a budding scientist or engineer...
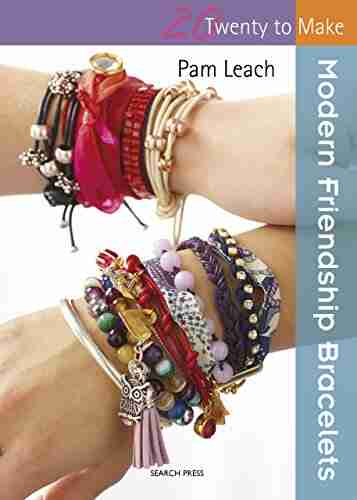

Discover the Coolest and Trendiest Friendship Bracelets -...
Friendship bracelets have...
Light bulbAdvertise smarter! Our strategic ad space ensures maximum exposure. Reserve your spot today!
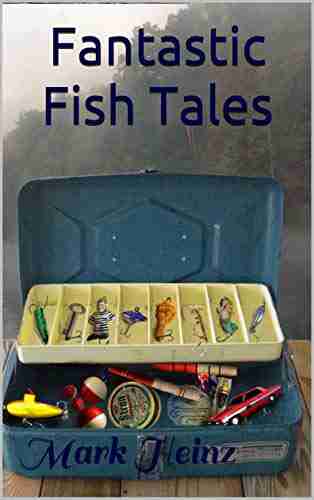

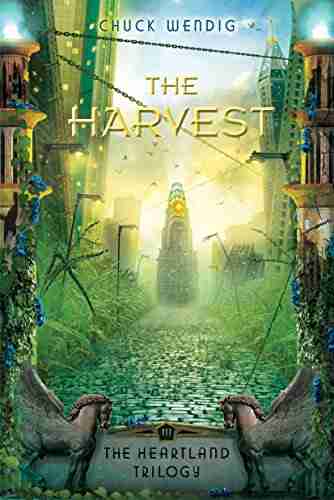

- Levi PowellFollow ·16.1k
- Henry HayesFollow ·12.1k
- Anthony BurgessFollow ·5.5k
- Nathan ReedFollow ·12.4k
- Gustavo CoxFollow ·3.6k
- Herbert CoxFollow ·13k
- Gage HayesFollow ·3.9k
- Walt WhitmanFollow ·16k